5、场景应用——电商平台:浏览记录收集功能
事件/日志收集系统是大中型软件不得不面对的话题。目前第三方业务系统对 事件/日志收集系统 的集成思路主要有两大类:侵入式收集方案和非侵入式收集方案。侵入式收集方案,是指任何需要使用事件/日志收集系统的第三方系统,都需要做有针对的编码工作,这个编码工作或者是新增代码用于调用 事件/日志收集系统 提供的客户端API,又或者是修改已有的代码,以便适应事件/日志收集系统的调用特性。
侵入式方案又分为半侵入式和全侵入式。由于第三方系统的代码结构本身存在问题,所以一旦需要集成 事件/日志收集系统(或者任何其他第三方系统),就会造成业务处理过程改变。相反,由于需求变动导致的业务代码变动,也会牵扯到任意的第三方系统集成代码的改变。这样的集成方式就是全侵入式的。出现这种的情况,是第三方系统所选择的技术方案和业务系统本身的工程结构问题共同造成的。
很显然,全侵入式的方案是一种错误的设计实践,在日常的设计工作中是要尽量避免。半侵入式方案比全侵入方案要好很多:虽然第三方系统会针对 事件/日志收集系统 做一定的代码改造,但是由于第三方系统的结构清晰,所以这部分代码和第三方系统原有的业务代码是完全分离的,只需要改造一次就可一直使用。也不会对第三方系统既有的业务处理过程产生任何影响,反之第三方系统由于需求变化产生的业务代码变化也不会影响 事件/日志收集系统 的客户端集成代码变化。
事件/日志收集系统 另外一种设计方案是非侵入式的。即业务系统在集成 事件/日志收集系统时,不需要为这件事情专门引入新的代码或者修改已有代码。业务系统的开发人员甚至完全不知道(也不必知道)自己的系统集成了 事件/日志收集系统,仅通过配置一些参数文件的方式就可完成集成工作。
我们将通过包括本文章在内的2-3篇文章的篇幅,利用已经学习过的技术知识向大家介绍事件/日志收集系统的半侵入方案和非侵入式方案。当然中间还会穿插一些新技术的介绍,比如Apache Flume。
5-1、场景说明
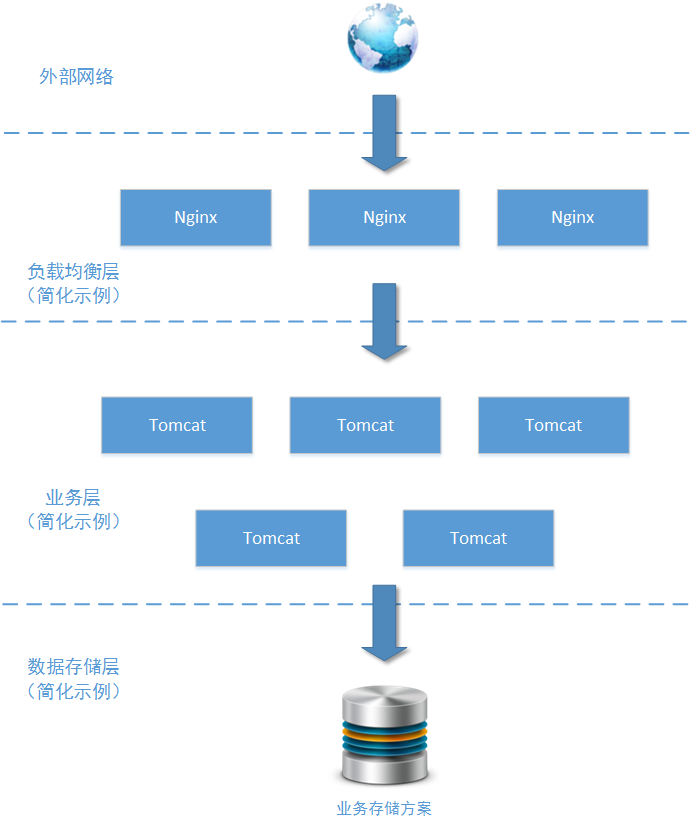
这是一个日均200万PV的中型电商网站的一个系统模块:商品详情模块。这个模块用于(且只用于)向用户展示商品详情、展示商品价格走势。上图所示中,该模块只列举了使用的主要技术组件,毕竟这个实例场景不是为了讨论这些技术组件本身。由于网站业务的发展需要,需要在这个模块加入用户操作的统计分析功能,对用户“点击查看订单详情”、“点击查看商品价格走势”等操作动作进行事件/日志收集。
为什么要对这些操作进行统计呢?因为这些数据能够说明某一个用户在一个特定的时间段对哪些商品感兴趣,预计对哪些(或哪一类)商品会产生购买订单。借助后端的数据分析手段,还能知晓某一类用户对哪一类商品感兴趣的概率配比。所以这些商品详情查看的操作日志特别有商业价值。
日均200万PV是一个什么概念呢?这么说吧,翼支付(bestpay.com.cn)的日均PV在34万左右,汽车之家(autohome.com.cn)的日均PV在100万左右,折800(zhe800.com)的日均PV在600万,携程在线(ctrip.com)日均PV1200万,京东(jd.com)日均PV3.7亿,淘宝(taobao.com)日均6.4亿(以上数据均来自alexa.cn)……
PV是Page View的简称,即一次页面的完整打开算作一次PV。PV的统计中,这次页面访问“是由那个访问者发起的”并不会对统计结果构成直接影响,也就是说即使是同一个访问者连续两次打开同一个页面,也会算作PV=2。这里要注意的另外一个问题是,由于在浏览器页面上会有很多访问连接(例如:多个图片连接、多个AJAX请求等),所以一次PV可能会包含多次对服务端的请求。
作为架构师,您的工作职责就是为这个日志记录系统设计一个易于业务扩展和技术扩展的软件架构。所谓易于业务扩展是指:也许在未来的某个日子不只是“商品详情模块”会集成本系统,用户中心模块也会集成本系统又或者订单子系统也会集成本系统,您设计的日志收集子系统应该可以在未来被这些子系统轻松集成,而不需要修改 日志收集系统 的任何代码(目标子系统也只需要修改极少的代码,甚至不修改代码)。
所谓技术扩展主要是说“日志收集系统”支撑的数据吞吐量可以进行可靠的横向扩展,而不需要停止服务或者要求业务系统进行改动,毕竟要相应考虑以上业务扩展中所描述的多种业务系统可可以进行集成的问题。另外,由于未来很多第三方系统都需要进行集成,作为架构师的您不可能知晓这些第三方系统会使用的是什么编程语言,更不可能限制第三方系统必须使用哪些编程语言。所以在进行 事件/日志收集系统 的设计时,需要考虑一种兼容各种编程语言的设计思路。
5-2、解决方案一:半侵入式方案
我们先来看看此问题的第一种解决方案。如果您确定将要集成 事件/日志收集系统 的所有第三方业务系统都有良好的代码结构(当然实际工作这种情况不太可能),那么为这些第三方系统提供相应编程语言的客户端API,就是一个可选择的方案:
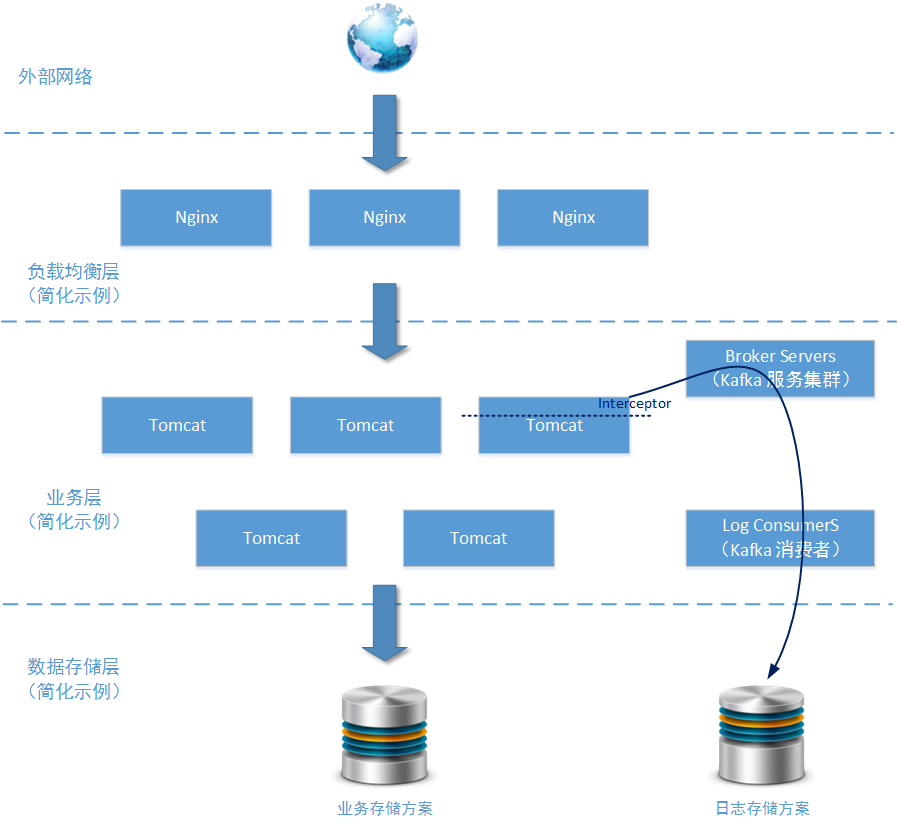
所有操作日志在业务系统上使用过滤器/拦截器的方式对需要进行收集的访问请求进行拦截。分离出访问地址、访问用户、访问时间等重要信息后,将其作为Kafka消息发送给Kafka Brokers 集群。这些信息将最终到达由若干Kafka Consumers节点组成的处理服务,并使用适当的存储方案直接存储到连续文件中(存储到HBase、Cassandra这样的数据库中也行,具体看这些日志数据将会被用于怎样的分析场景)。
5-2-1、设计重点说明
上图中,主要的展示目的是事件/日志收集系统在业务系统端是怎样被集成的。所以关于事件/日志收集系统的结构就画得比较简单。只给出了两个区块“Broker Servers”和“Log Consumers”,下面我们重点分析一下本方案中的 事件/日志收集系统 的核心结构:
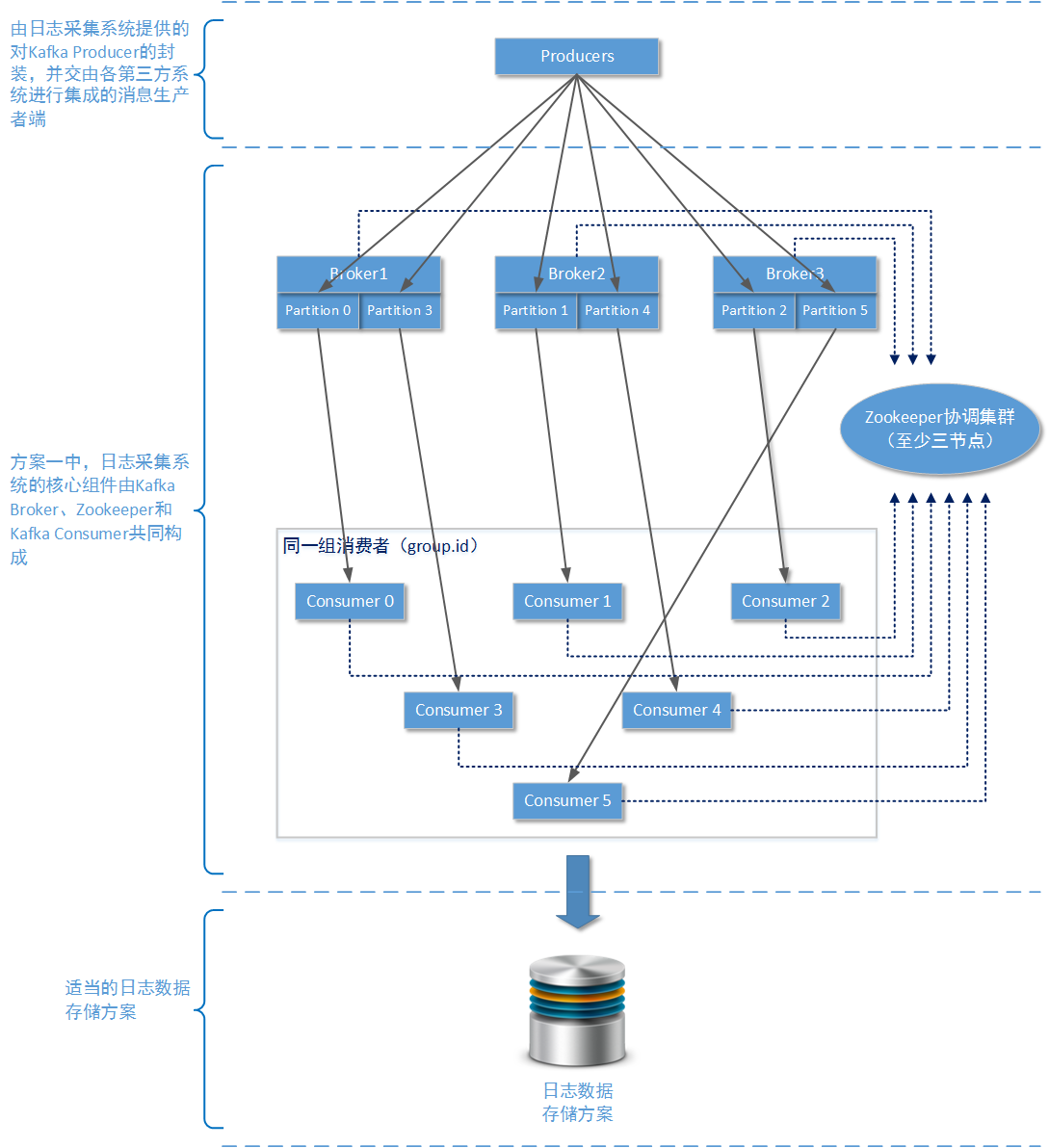
在方案一种,我们主要使用单纯以Apache Kafka为核心的消息队列解决方案。
使用Apache Kafka时,如果您只是用一个zookeeper服务节点,整个集群也能正常工作。但是由于单个节点的zookeeper服务基本上没有容错能力,一旦单个zookeeper节点由于各种原因宕机,整个Apache Kafka集群就会崩溃。所以建议在生产环境下,至少为zookeeper服务准备三个服务节点,这样当某个zookeeper服务节点出现故障整个Apache Kafka服务还可以正常运行(三个节点得zookeeper服务最多允许一个节点发生故障)。
在生产环境下为了保证整个Kafka集群的稳定,请至少使用3个Brokers物理节点。考虑到后期多个业务系统可能会使用事件/日志收集系统,那么可以在首次设计时将Brokers设定为5个Brokers物理节点。在之前的文章中我们已经详细介绍过Apache Kafka的工作原理,Brokers越多、Topic的分区(partition)越多,整个Apache Kafka集群的稳定性和吞吐量就越好。
再说明一下其中的复制因子数量设置,复制因子对消息可靠性有直接影响,并且在设定为强一致性工作模式下也会对消息吞吐量产生影响。由于我们使用Kafka主要是为了接收/发送日志数据,在运行过程中丢失一两条日志是可以容忍的错误。所以建议设置复制因子数量为 “Brokers数量 / 2 + 1”,并且在生产者端使用“弱一致性”发送模式,即acks == 1。
为了区分日志数据来自于哪一个业务系统,可以专门为不同的业务系统设置独立的Topic。分区数量最好为Brokers数量的整数倍,这样才能确保在每一个物理节点在硬件配置相同的情况下,能够很好的均分吞吐量压力。具体来说,由于我们在生产环境采用了5个Brokers物理节点,那么每一个Topic的分区数量最好为5的整数倍,例如您可以设置分区数量为10。
既然设置分区数量为10,那么同一个消费者组的消费者数量最科学的值也是10。因为Kafka集群中存在同一个分区的数据在同一时间最多被一个消费者所消费的限制,所以如果存在第11个消费者,它也只能处于备用等待状态。待到某个消费者出现问题时,再由第11个消费者进行顶替。实际上在 事件/日志采集系统 中这样的Apache Kafka集群规模,已经完全可以应付日均200万PV的网站系统对日志采集工作的吞吐量要求了。
日志数据的分析手段一般有两种:实时分析和离线分析。所谓实时分析是说分析服务在接收到日志数据后,立即对产生的后果进行计算并将分析结果记录在某个存储方案上。Apache Storm、Apache Spark都是常用的实时分析系统,不过在本专题中并不会对Apache Storm或者Apache Spark进行详细介绍,毕竟这属于另一个知识领域了(数据分析以后会有专门的专题进行讲解。当然,读者也可以认为作者压根不知道)。实时分析在生产环境中有很多应用,例如根据用户的上线/下线日志对用户的在线数量进行实时统计;根据商品的点击情况,对商品的查看数量进行实时统计;根据用户的页面跳转情况实时形成用户浏览轨迹地图。
日志数据的另一种分析手段是离线分析。即分析服务在接收到原始日志数据后并不做任何处理,只是将原始数据按照预定的格式(又或者就是数据本来的格式)存储到某个位置。当某个时间周期到来或者具体的事件被触发时,再由其他软件对这些数据进行分析。Apache Hadoop/Cloudera Hadoop就是常用的离线分析工具。您可以通过某种手段,将原始的日志信息存储在HDFS文件系统上,以便Hadoop进行离线分析。离线分析在实际生产环境中也有很多应用,例如按照用户的商品浏览情况分析用户的购买趋势、利用商品关键词进一步分析适合销售的用户群体、利用商品库存和价格走势预测最佳补货时机。
无论是实时分析还是离线分析Kafka的下层系统(组件)都需要做存储操作。例如您可以直接使用Kafka的消费者将消息写入Cassandra集群、可以将Kafka接受到的数据作为Apache Strom 的Spout,直接送入Strom的管道(进行实时分析)。如果您要将日志写入HDFS文件系统,则可以直接使用Flume(这个在后续的示例方案中会讲到)。不过,请别做愚蠢的事情:不要将日志数据送入任何关系型数据库。
在接下来的方案演示中我们假定业务系统基于JAVA,并且已经集成了Spring框架。由于在本方案中我们使用了过滤器(Filters)/拦截器(Interceptor)隔离操作日志,所以业务服务中怎样进行业务层和数据层的处理本方案可以不必过多关注:
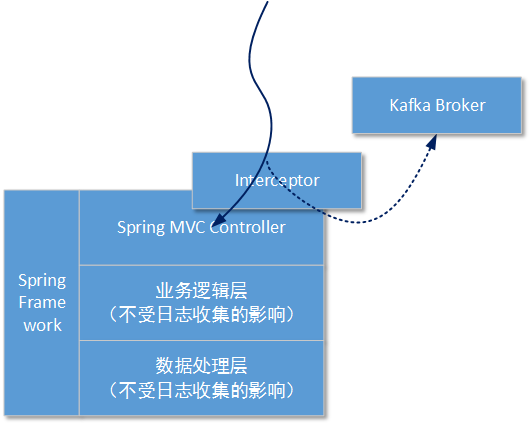
这样做的好处是,可以将对日志的拦截操作在执行真正的业务操作前进行隔离,业务处理代码不需要关心在这之前都有多少层拦截,只需要按照原有的处理逻辑执行就行。
5-2-2、编码过程:生产者和业务系统集成
演示的业务工程将使用Spring-MVC组件,所以如果您需要查看演示效果,请在工程中导入Spring-MVC组件(V3.2.X的版本都行):
<dependency>
<groupId>org.springframework</groupId>
<artifactId>spring-webmvc</artifactId>
<version>3.2.10.RELEASE</version>
</dependency>
为了让更多的读者理解整个过程,我们首先来看一下这个拦截器的使用方式:
package templateSSHProject.controller;
import javax.servlet.http.HttpServletRequest;
import javax.servlet.http.HttpServletResponse;
import org.springframework.stereotype.Controller;
import org.springframework.web.bind.annotation.RequestMapping;
import templateSSHProject.controller.kafkaproducer.LogAnnotation;
/**
* spring MVC组件搭建的http控制层
* @author yinwenjie
*/
@Controller
@RequestMapping("/")
public class UserController extends BaseController {
/**
* 查询所有用户信息,但是不包括关联信息
* @param request
* @param response
*/
@LogAnnotation
@RequestMapping("/queryAllUser")
public void queryAllUserWithoutParent(HttpServletRequest request , HttpServletResponse response) {
}
}
- 1
- 2
- 3
- 4
- 5
- 6
- 7
- 8
- 9
- 10
- 11
- 12
- 13
- 14
- 15
- 16
- 17
- 18
- 19
- 20
- 21
- 22
- 23
- 24
- 25
- 26
- 27
- 28
- 29
- 30
- 31
- 32
- 33
以上代码片段是一个基于Spring-MVC组件编写的Http Controller层的类,名叫UserController(当然这个类是被Spring Ioc容器托管了)。在浏览器上我们可以使用 http://ip:port/queryAllUser 这样的URL访问到queryAllUserWithoutParent方法。
请注意在queryAllUserWithoutParent方法上,我们使用了一个“@LogAnnotation”自定义注解。这个注解表示:当方法被调用时,这个业务系统需要向 事件/日志收集系统 发送日志信息。
“@LogAnnotation”注解的定义非常简单,毕竟它只是一个标识,并不是整个结构能够运行起来的核心动力。
package templateSSHProject.controller.kafkaproducer;
import java.lang.annotation.ElementType;
import java.lang.annotation.Retention;
import java.lang.annotation.RetentionPolicy;
import java.lang.annotation.Target;
/**
* 拦截标识注解。使用这个注解的方法说明需要向 事件/日志服务发送消息
* @author yinwenjie
*/
@Target(ElementType.METHOD)
@Retention(RetentionPolicy.RUNTIME)
public @interface LogAnnotation {
/**
* 您可以根据自己的需要,在这个注解中加入各种属性
* @return
*/
public String message() default "";
}
- 1
- 2
- 3
- 4
- 5
- 6
- 7
- 8
- 9
- 10
- 11
- 12
- 13
- 14
- 15
- 16
- 17
- 18
- 19
- 20
- 使用Spring-MVC的Interceptor拦截器,对HTTP请求进行拦截
好了,为了让以上的代码能够运行起来。我们需要使用基于Spring-MVC的Interceptor拦截器,对HTTP请求进行拦截。让它在正式到达(执行)queryAllUserWithoutParent方法前,能够先被拦截器预先处理。首先我们需要定义一个拦截器,如下所示:
package templateSSHProject.controller.kafkaproducer;
import java.lang.reflect.Method;
import java.util.Date;
import javax.servlet.http.HttpServletRequest;
import javax.servlet.http.HttpServletResponse;
import org.springframework.beans.factory.annotation.Autowired;
import org.springframework.web.method.HandlerMethod;
import org.springframework.web.servlet.handler.HandlerInterceptorAdapter;
import test.interrupter.producer.ProducerService;
/**
* 日志拦截器。一定注意,拦截器是基于Spring MVC的。<br>
* 如果您使用的是Struts组件,那么就应该使用Struts提供的拦截器;
* @author yinwenjie
*/
public class LogMethodInterceptor extends HandlerInterceptorAdapter {
/**
* 由 事件/日志 系统提供的客户端工具包,
* 并且使用spring进行代理的消息生产者服务对象。
* 而且已经在spring配置中使用了singleton进行标记,说明全系统只有一个生产者服务对象
*/
@Autowired
private ProducerService producerService;
@Override
public boolean preHandle(HttpServletRequest request, HttpServletResponse response, Object handler) throws Exception {
if (handler == null || !(handler instanceof HandlerMethod)) {
return true;
}
HandlerMethod handlerMethod = (HandlerMethod)handler;
Method method = handlerMethod.getMethod();
LogAnnotation logAnnotation = method.getAnnotation(LogAnnotation.class);
if(logAnnotation == null) {
return true;
}
Class<?> declaringClass = method.getDeclaringClass();
String declaringClassName = declaringClass.getName();
String methodName = method.getName();
String message = declaringClassName + ":" + methodName + "[" + new Date().getTime() + "]";
this.producerService.senderMessage(message);
return true;
}
}
- 1
- 2
- 3
- 4
- 5
- 6
- 7
- 8
- 9
- 10
- 11
- 12
- 13
- 14
- 15
- 16
- 17
- 18
- 19
- 20
- 21
- 22
- 23
- 24
- 25
- 26
- 27
- 28
- 29
- 30
- 31
- 32
- 33
- 34
- 35
- 36
- 37
- 38
- 39
- 40
- 41
- 42
- 43
- 44
- 45
- 46
- 47
- 48
- 49
- 50
- 51
- 52
- 53
- 54
- 55
- 56
- 57
- 58
- 59
- 60
- 61
所有的Spring-MVC Interceptor都要继承一个父类:org.springframework.web.servlet.handler.HandlerInterceptorAdapter,当然Interceptor也是被Spring-Ioc容器托管的。为了使用Interceptor,您需要在配置文件中加入相应的信息:
<mvc:interceptors>
<bean class="templateSSHProject.controller.kafkaproducer.LogMethodInterceptor"></bean>
</mvc:interceptors>
在HandlerInterceptorAdapter父类中,我们可以按照自身的需要选择性的重写preHandle方法、postHandle方法、afterCompletion方法或者afterConcurrentHandlingStarted方法。从这些方法名称就可以明白这些方法所代表的含义。这里我们选择重载其中的preHandle预处理方法。
请注意HandlerInterceptorAdapter类中定义的对象“private ProducerService producerService”。这个对象就是由 事件/日志收集系统提供的JAVA 客户端开发包中的主要服务类。第三方业务系统需要使用这个服务类和 事件/日志收集系统 进行通讯。
- 客户端开发包中的ProducerService定义和实现:
以下是生产者接口定义
package test.interrupter.producer;
/**
* 生产者服务
* @author yinwenjie
*/
public interface ProducerService {
/**
* 初始化kafka生产端的配置信息
*/
public void init();
/**
* 向kafka brokers发送消息
* @param message
*/
public void sendeMessage(String message);
}
- 1
- 2
- 3
- 4
- 5
- 6
- 7
- 8
- 9
- 10
- 11
- 12
- 13
- 14
- 15
- 16
- 17
- 18
以下是生产者接口实现:
package test.interrupter.producer;
import java.util.Properties;
import org.apache.commons.lang.StringUtils;
import kafka.javaapi.producer.Producer;
import kafka.producer.KeyedMessage;
import kafka.producer.ProducerConfig;
/**
* 生产者服务实现
* @author yinwenjie
*/
public class ProducerServiceImpl implements ProducerService {
/**
* kafka的brokers列表
*/
private String brokers;
/**
* acks的值,只能有三种-1、0还有1
*/
private Integer required_acks = 0;
/**
* 请求超时间,默认为1000l
*/
private Long request_timeout = 1000l;
/**
* kafka主服务对象
*/
private Producer<byte[], byte[]> producer;
/**
* 分区数量
*/
private Integer partitionNumber;
public void init() {
if(StringUtils.isEmpty(this.brokers)) {
throw new RuntimeException("至少需要指定一个broker的位置");
}
if(this.required_acks != 0 && this.required_acks != 1
&& this.required_acks != -1) {
throw new RuntimeException("错误的required_acks值!");
}
if(this.partitionNumber <= 0) {
throw new RuntimeException("partitionNumber至少需要有1个");
}
Properties props = new Properties();
props.put("metadata.broker.list", this.brokers);
props.put("producer.type", "sync");
props.put("request.required.acks", this.required_acks.toString());
props.put("request.timeout.ms", this.request_timeout.toString());
ProducerConfig config = new ProducerConfig(props);
this.producer = new Producer<byte[], byte[]>(config);
}
public void sendeMessage(String message) {
byte[] messageContext = message.getBytes();
KeyedMessage<byte[], byte[]> keyedMessage = new KeyedMessage<byte[], byte[]>("MessageTopic", messageContext , null , messageContext);
this.producer.send(keyedMessage);
}
/**
* @return the brokers
*/
public String getBrokers() {
return brokers;
}
/**
* @param brokers the brokers to set
*/
public void setBrokers(String brokers) {
this.brokers = brokers;
}
/**
* @return the required_acks
*/
public Integer getRequired_acks() {
return required_acks;
}
/**
* @param required_acks the required_acks to set
*/
public void setRequired_acks(Integer required_acks) {
this.required_acks = required_acks;
}
/**
* @return the request_timeout
*/
public Long getRequest_timeout() {
return request_timeout;
}
/**
* @param request_timeout the request_timeout to set
*/
public void setRequest_timeout(Long request_timeout) {
this.request_timeout = request_timeout;
}
/**
* @return the partitionNumber
*/
public Integer getPartitionNumber() {
return partitionNumber;
}
/**
* @param partitionNumber the partitionNumber to set
*/
public void setPartitionNumber(Integer partitionNumber) {
this.partitionNumber = partitionNumber;
}
}
- 1
- 2
- 3
- 4
- 5
- 6
- 7
- 8
- 9
- 10
- 11
- 12
- 13
- 14
- 15
- 16
- 17
- 18
- 19
- 20
- 21
- 22
- 23
- 24
- 25
- 26
- 27
- 28
- 29
- 30
- 31
- 32
- 33
- 34
- 35
- 36
- 37
- 38
- 39
- 40
- 41
- 42
- 43
- 44
- 45
- 46
- 47
- 48
- 49
- 50
- 51
- 52
- 53
- 54
- 55
- 56
- 57
- 58
- 59
- 60
- 61
- 62
- 63
- 64
- 65
- 66
- 67
- 68
- 69
- 70
- 71
- 72
- 73
- 74
- 75
- 76
- 77
- 78
- 79
- 80
- 81
- 82
- 83
- 84
- 85
- 86
- 87
- 88
- 89
- 90
- 91
- 92
- 93
- 94
- 95
- 96
- 97
- 98
- 99
- 100
- 101
- 102
- 103
- 104
- 105
- 106
- 107
- 108
- 109
- 110
- 111
- 112
- 113
- 114
- 115
- 116
- 117
- 118
- 119
- 120
- 121
- 122
- 123
- 124
- 125
- 126
- 127
- 128
- 129
- 130
- 131
- 132
作为使用java开发的业务系统,至少有两种方式使用上一小节中定义的生产者服务接口。一种是在业务系统中的过滤器/拦截器中“new”这个类,然后手动调用初始化方法,最后再调用sendMessage方法;还好,我们示例中的业务系统使用了Spring容器,所以我们可以使用第二种方法:将生产者服务注入容器,然后直接在过滤器/拦截器中调用sendMessage方法。
您需要在业务系统的配置栏目中加入新的bean定义:
......
<bean id="producerService" class="test.interrupter.producer.ProducerServiceImpl" init-method="init" scope="singleton">
<property name="brokers">
<value>${kafka.producer.brokers }</value>
</property>
<property name="partitionNumber">
<value>${kafka.producer.partitionNumber }</value>
</property>
<property name="required_acks">
<value>${kafka.producer.required_acks }</value>
</property>
</bean>
......
- 1
- 2
- 3
- 4
- 5
- 6
- 7
- 8
- 9
- 10
- 11
- 12
- 13
- 14
- 15
- 16
- 17
和kafka brokers通讯的主要参数我们放置在一个properties文件中,方便部署时进行更改(kafka.properties):
kafka.producer.brokers=192.168.61.138:9092,192.168.61.139:9092
kafka.producer.partitionNumber=10
kafka.producer.required_acks=1
以上就是业务系统需要使用消息生产服务所进行的更改,以及生产服务自身是如何定义的。可以看到,这种半侵入式的集成方式下,我们确实需要为集成 事件/日志收集系统 做很多的配置、编码工作。好的一方面是这些工作并不会影响原有的业务系统处理过程。
5-2-3、是否使用Spring Integration-Kafka
Spring Integration(http://projects.spring.io/spring-integration/)是依赖Spring核心框架进行工作的一套扩展组件。通过这套组件开发人员可以方便的在应用工程上集成第三方中间件技术,例如使用Spring Integration-Redis集成对外部Redis服务的调用、使用Spring Integration-FTP 集成对外部FTP服务的调用、使用Spring Integration-Kafka 集成对外部Kafka服务的调用。
Spring Integration非常轻量、易于测试、入门文档较全、几乎没有使用门槛,只要知道Spring框架的基本使用方式就行。使用Spring Integration来实现对外部中间件服务的调用,大多数情况下比“自己编写”的解决方式都要好。
虽然Spring Integration框架非常好用,也确实节省了相当的集成工作,减少了错误调用的风险。但可能要让各位读者要失望了:因为开发人员不能确定,将要集成 事件/日志收集系统 的所有业务系统都是基于Spring框架进行构建。所以在这样的背景下,提供给基于JAVA(或者其扩展语言:Groovy、Scala)业务系统使用生产者服务,不应该和Spring形成强依赖关系,以保证在没有使用Spring框架的JAVA业务系统上也实现生产者服务的集成。
在本小节中我们展示生产者端的示例代码,并没有经过优化。例如虽然通过Spring框架分离了业务代码和日志发送代码,但是一旦http请求到来,这些代码还是会在同一个线程运行。那么如果出现由于远端的Kafka服务拥堵导致的生产者发送缓慢的情况,就会影响到业务服务中对业务请求的处理速度。
要解决这个问题,可以在业务系统中为生产者服务开辟专门的处理线程池。利用线程池的BlockingQueue队列存储待发送的日志消息,利用独立线程进行日志消息的发送。不过这个解决办法并不是最好的,只能算是一个办法。因为生成者最终还是会占用业务系统紧张的JVM资源,还是会在将自身的异常状况转嫁给业务系统,在后面的方案中笔者还会提到这个问题。
5-2-4、编码过程:消费者端
存在于 事件/日志收集系统内部的 Kafka消息消费者端的代码工作也是非常简单的。Kafka消息消费者的工作只是用来接收这些这些日志数据并且使用“适当的存储方案” 将这些消息存储起来(或者送入另一处理组件,例如Strom)
下面给出一段可用的消息消费者端的代码:
- 一个ConsumerThread对象就代表一个消费者:
package com.test.logservice;
import org.apache.commons.logging.Log;
import org.apache.commons.logging.LogFactory;
import kafka.consumer.ConsumerIterator;
import kafka.consumer.KafkaStream;
import kafka.message.MessageAndMetadata;
/**
* 消息消费线程
* @author yinwenjie
*/
public class ConsumerThread implements Runnable {
private KafkaStream<byte[], byte[]> stream;
/**
* 日志
*/
private static Log LOGGER = LogFactory.getLog(ConsumerThread.class);
@Override
public void run() {
ConsumerIterator<byte[], byte[]> iterator = this.stream.iterator();
while(iterator.hasNext()) {
MessageAndMetadata<byte[], byte[]> message = iterator.next();
int partition = message.partition();
String topic = message.topic();
String messageT = new String(message.message());
ConsumerThread.LOGGER.info("接收到: " + messageT + "来自于topic:[" + topic + "] + 第partition[" + partition + "]");
}
}
/**
* @param stream the stream to set
*/
public void setStream(KafkaStream<byte[], byte[]> stream) {
this.stream = stream;
}
}
- 1
- 2
- 3
- 4
- 5
- 6
- 7
- 8
- 9
- 10
- 11
- 12
- 13
- 14
- 15
- 16
- 17
- 18
- 19
- 20
- 21
- 22
- 23
- 24
- 25
- 26
- 27
- 28
- 29
- 30
- 31
- 32
- 33
- 34
- 35
- 36
- 37
- 38
- 39
- 40
- 41
- 42
- 43
- 44
- 45
- 46
- 47
- 48
- 49
- 50
- 51
- 52
- 53
- KafkaConsumerLauncher基于spring框架连接zk并且启动消息消费者:
package com.test.logservice;
import java.util.HashMap;
import java.util.List;
import java.util.Map;
import java.util.Properties;
import java.util.concurrent.ThreadPoolExecutor;
import org.springframework.context.ApplicationContext;
import org.springframework.context.ApplicationListener;
import org.springframework.context.event.ContextRefreshedEvent;
import kafka.consumer.Consumer;
import kafka.consumer.ConsumerConfig;
import kafka.consumer.KafkaStream;
import kafka.javaapi.consumer.ConsumerConnector;
/**
* 这是Kafka的topic消费者
* @author yinwenjie
*/
public class KafkaConsumerLauncher implements ApplicationListener<ContextRefreshedEvent> {
/**
* zookeeper连接地址串
*/
private String zookeeper_connects;
/**
* zookeeper连接超时事件
*/
private Long zookeeper_timeout;
/**
* 分区数量
*/
private Integer consumerNumber;
/**
* 消息消费者处理线程池。
* 每一个消费者都是线程池中的一个线程<br>
* 且线程池中线程数量就是分区数量
*/
private ThreadPoolExecutor consumerPool;
@Override
public void onApplicationEvent(ContextRefreshedEvent event) {
ApplicationContext ac = event.getApplicationContext();
if(ac.getParent() == null) {
this.startConsumerStream(ac);
}
}
/**
* 开启消费者线程
* @param context
*/
public void startConsumerStream(ApplicationContext context) {
Properties props = new Properties();
props.put("zookeeper.connect", this.zookeeper_connects);
props.put("zookeeper.connection.timeout.ms", this.zookeeper_timeout.toString());
props.put("group.id", "consumerGroup");
ConsumerConfig consumerConfig = new ConsumerConfig(props);
ConsumerConnector consumerConnector = Consumer.createJavaConsumerConnector(consumerConfig);
HashMap<String, Integer> map = new HashMap<String, Integer>();
String topicName = "MessageTopic";
map.put(topicName, this.consumerNumber);
Map<String, List<KafkaStream<byte[], byte[]>>> topicMessageStreams = consumerConnector.createMessageStreams(map);
List<KafkaStream<byte[], byte[]>> streamList = topicMessageStreams.get(topicName);
for(int index = 0 ; index < streamList.size() ; index++) {
KafkaStream<byte[], byte[]> stream = streamList.get(index);
ConsumerThread consumerThread = (ConsumerThread)context.getBean("consumerThread");
consumerThread.setStream(stream);
this.consumerPool.submit(consumerThread);
}
}
/**
* @return the zookeeper_connects
*/
public String getZookeeper_connects() {
return zookeeper_connects;
}
/**
* @param zookeeper_connects the zookeeper_connects to set
*/
public void setZookeeper_connects(String zookeeper_connects) {
this.zookeeper_connects = zookeeper_connects;
}
/**
* @return the zookeeper_timeout
*/
public Long getZookeeper_timeout() {
return zookeeper_timeout;
}
/**
* @param zookeeper_timeout the zookeeper_timeout to set
*/
public void setZookeeper_timeout(Long zookeeper_timeout) {
this.zookeeper_timeout = zookeeper_timeout;
}
/**
* @return the consumerNumber
*/
public Integer getConsumerNumber() {
return consumerNumber;
}
/**
* @param consumerNumber the consumerNumber to set
*/
public void setConsumerNumber(Integer consumerNumber) {
this.consumerNumber = consumerNumber;
}
/**
* @return the consumerPool
*/
public ThreadPoolExecutor getConsumerPool() {
return consumerPool;
}
/**
* @param consumerPool the consumerPool to set
*/
public void setConsumerPool(ThreadPoolExecutor consumerPool) {
this.consumerPool = consumerPool;
}
}
- 1
- 2
- 3
- 4
- 5
- 6
- 7
- 8
- 9
- 10
- 11
- 12
- 13
- 14
- 15
- 16
- 17
- 18
- 19
- 20
- 21
- 22
- 23
- 24
- 25
- 26
- 27
- 28
- 29
- 30
- 31
- 32
- 33
- 34
- 35
- 36
- 37
- 38
- 39
- 40
- 41
- 42
- 43
- 44
- 45
- 46
- 47
- 48
- 49
- 50
- 51
- 52
- 53
- 54
- 55
- 56
- 57
- 58
- 59
- 60
- 61
- 62
- 63
- 64
- 65
- 66
- 67
- 68
- 69
- 70
- 71
- 72
- 73
- 74
- 75
- 76
- 77
- 78
- 79
- 80
- 81
- 82
- 83
- 84
- 85
- 86
- 87
- 88
- 89
- 90
- 91
- 92
- 93
- 94
- 95
- 96
- 97
- 98
- 99
- 100
- 101
- 102
- 103
- 104
- 105
- 106
- 107
- 108
- 109
- 110
- 111
- 112
- 113
- 114
- 115
- 116
- 117
- 118
- 119
- 120
- 121
- 122
- 123
- 124
- 125
- 126
- 127
- 128
- 129
- 130
- 131
- 132
- 133
- 134
- 135
- 136
- 137
- 138
- 139
- 140
- 141
- 142
- 143
- 144
- 145
- 146
- 147
- 148
KafkaConsumerLauncher中我们一共为名叫“MessageTopic”的Topic创建了10个消费者。就像讲解Kafka特性时提到的那样:消费者数量不要小于Topic的分区数量,也可以多出一些消费者数量作为备用。这样才能保证每一个分区都有一个对应的消费者进行消费。如果在您的集群中,设计了5个消费节点作为消费者,那么也可以为每一个消费者应用程序创建两个消费者,这样一共也有10个消费者了。
另外注意,在KafkaConsumerLauncher中我们使用了一个线程池对象consumerPool,并且使用了Spring框架进行了注入;我们创建具体的消费者线程也是依托于Spring框架完成的,所以才会有“context.getBean”这样的语句。它们的xml配置情况如下:
<bean id="kafkaConsumerLauncher" class="com.test.logservice.KafkaConsumerLauncher" scope="singleton">
<property name="consumerNumber" value="10"></property>
<property name="consumerPool" ref="consumerPool"></property>
<property name="zookeeper_connects" value="192.168.61.138:2181"></property>
<property name="zookeeper_timeout" value="10000"></property>
</bean>
<bean id="consumerPool" scope="singleton" class="java.util.concurrent.ThreadPoolExecutor">
<constructor-arg value="10" type="int"></constructor-arg>
<constructor-arg value="10" type="int"></constructor-arg>
<constructor-arg value="10000" type="long"></constructor-arg>
<constructor-arg value="MILLISECONDS" type="java.util.concurrent.TimeUnit"></constructor-arg>
<constructor-arg ref="threadCacheQueue"></constructor-arg>
</bean>
<bean id="threadCacheQueue" class="java.util.concurrent.SynchronousQueue"></bean>
<bean id="consumerThread" class="com.test.logservice.ConsumerThread" scope="prototype"></bean>
- 1
- 2
- 3
- 4
- 5
- 6
- 7
- 8
- 9
- 10
- 11
- 12
- 13
- 14
- 15
- 16
- 17
- 18
- 19
- 20
- 21
- 22
- 23
- 24
- 25
- 26
- 27
- 28
- 29
- 30
=========================
(接下文)
所有评论(0)